

Economics and Business
Quarterly Reviews
ISSN 2775-9237 (Online)


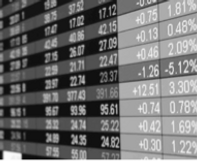




Published: 25 July 2022
Predicting Household Resilience Before and During Pandemic with Classifier Algorithms
Ndari Surjaningsih, Hesti Werdaningtyas, Faizal Rahman, Romadhon Falaqh
Central Bank of Indonesia, Indonesia
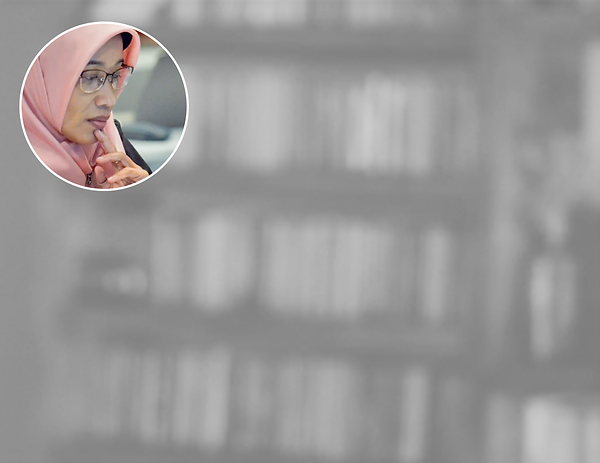
Download Full-Text Pdf

10.31014/aior.1992.05.03.437
Pages: 75-81
Keywords: Default Event, Household Resilience, Vulnerability, Machine Learning
Abstract
One of the lessons learned from the global financial crisis in 2008 was raising attention to monitoring and maintaining household vulnerability, particularly household credit risk, by using the default rate as the indicator. The indicator would be worsening at the economic recession, likewise, recently happened caused by the pandemic. The default event has a complex nonlinearity relationship among the determinants. To tackle the complex relationship, this study suggests exploiting machine learning approach in modeling the probability of default, especially the individual and ensemble classifiers. Therefore, this study aims to investigate changes of the Indonesian household financial resilience before and during the pandemic, supported by the individual-level data of the Financial Information Service System. This study finds that the ensemble classifiers, notably extreme gradient boosting, have a more predominant performance than the individual classifiers. The best model, then has the feature importance analysis to identify the variable pattern in explaining the default event periodically which reveals the pattern changes before and during the pandemic. The cost of debt/repayment capability and the policy mix is significant in explaining the default event. At the same time, the project location feature weakens in discriminating the target class.
References
Albacete, N., & Lindner, P. (2013). Household Vulnerability in Austria–A Microeconomic Analysis Based on the Household Finance and Consumption Survey. Financial Stability Report, 57-73.
Alfaro, R., & Gallardo, N. (2012). The determinants of household debt default.
Baesens, B., Van Gestel, T., Viaene, S., Stepanova, M., Suykens, J., & Vanthienen, J. (2003). Benchmarking state-of-the-art classification algorithms for credit scoring. Journal of the operational research society, 54(6), 627-635. DOI: 10.1057/palgrave.JORS .2601545
Bahnsen, A. C., Aouada, D., & Ottersten, B. (2014, December). Example-dependent cost-sensitive logistic regression for credit scoring. In 2014 13th International conference on machine learning and applications (pp. 263-269). IEEE.
Barbaglia, L., Manzan, S., & Tosetti, E. (2020). Forecasting loan default in Europe with machine learning. Available at SSRN 3605449.
Bensic, M., Sarlija, N., & Zekic‐Susac, M. (2005). Modelling small‐business credit scoring using logistic regression, neural networks, and decision trees. Intelligent Systems in Accounting, Finance & Management: International Journal, 13(3), 133-150.
Brown, I., & Mues, C. (2012). An experimental comparison of classification algorithms for imbalanced credit scoring data sets. Expert Systems with Applications, 39(3), 3446-3453.
Chopra, A., & Bhilare, P. (2018). Application of ensemble models in credit scoring models. Business Perspectives and Research, 6(2), 129-141.
Crook, J., Edelman, D., & Thomas, L. (2007). Recent developments in consumer credit risk assessment. Eur. J. Oper. Res. 183, 1447–1465
Deng, Y., & Liu, P. (2009). In China's housing market, mortgage prepayment and default behaviour with embedded forward contract risks. The Journal of Real Estate Finance and Economics, 38(3), 214-240.
Feng, X., Xiao, Z., Zhong, B., Qiu, J., & Dong, Y. (2018). Appl. Soft Comput. (ISSN:1568-4946) 65, 139–151.
Heo, W., Lee, J. M., Park, N., & Grable, J. E. (2020). Using Artificial Neural Network techniques improves the description and prediction of household financial ratios. Journal of Behavioral and Experimental Finance, 25, 100273.
Jadhav, S., He, H., & Jenkins, K. (2018). Information gain directed genetic algorithm wrapper feature selection for credit rating. Appl. Soft Comput. (ISSN: 1568-4946).
Li, H., & Sun, J. (2013). Predicting business failure using an RSF-based case-based reasoning ensemble forecasting method. J. Forecasting 32, 180–192.
Marques, A., Garcia, V., & Sanchez, J. (2013). A literature review on the application of evolutionary computing to credit scoring. J. Oper. Res. Soc. 64, 1384–1399
Massaoudi, T. (2020). What You Should Know About Ensemble Learning. Retrieved from Towards Data Science: https://towardsdatascience.com/what-you-should-know-about-ensemble-learning-e92d4b3c3608
Shi, J., Zhang, S. Y., & Qiu, L. M. (2013). Credit scoring by feature-weighted support vector machines. J. Zhejiang Univ. Sci. C 14 (3), 197–204.
Teply, P., & Polena, M. (2020). Best classification algorithms in peer-to-peer lending. The North American Journal of Economics and Finance, 51. DOI: 10.1016/j.najef.2019.01.001
Trivedi, S. K. (2020). A study on credit scoring modelling with different feature selection and machine learning approaches. Technology in Society, 63, 101413.
Tsai, C. F., & Chen, M. L. (2010). Credit rating by hybrid machine learning techniques. Appl. Soft Comput. 10, 374–380.
Yeh, I. C., & Lien, C. H. (2009). The comparisons of data mining techniques for the predictive accuracy of probability of default of credit card clients. Expert Systems with Applications, 36(2), 2473-2480.
Yildrim, S. (2020). 11 Most Common Machine Learning Algorithms Explained in a Nutshell. Retrieved from Towards Data Science: https://towardsdatascience.com/11-most-common-machine-learning-algorithms-explained-in-a-nutshell-cc6e98df93be
Zekic-Susac, M., Sarlija, N., & Bensic, M. (2004, June). Small business credit scoring: a comparison of logistic regression, neural network, and decision tree models. In 26th International Conference on Information Technology Interfaces, 2004. (pp. 265-270). IEEE.