

Education Quarterly Reviews
ISSN 2621-5799

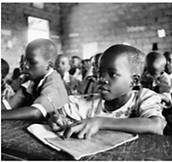





Published: 05 February 2025
Revolutionizing Fitness: The Intersection of Artificial Intelligence and Physical Activity
Simon Kormla Donkor
University of Education, Winneba

Download Full-Text Pdf

10.31014/aior.1993.08.01.556
Pages: 92-100
Keywords: Fitness, Artificial Intelligence (AI), Physical Activity, Pre-Service Teachers
Abstract
The rapid advancement of artificial intelligence (AI) technology is significantly reshaping the fitness industry, creating opportunities for enhanced personalization and effectiveness in exercise routines. This study explored the transformative impact of AI-powered wearables and smart fitness devices on individual fitness outcomes and overall physical activity levels. Utilizing a quantitative approach, the research involved a cross-sectional survey of 90 pre-service physical education students from the University of Education, Winneba, who provided insights into their experiences with AI technologies in fitness. The study employed a five (5) point Likert-scale questionnaire to assess respondents’ perceptions of AI's influence on their exercise regimens. Data was analyzed using means, standard deviation, independent samples t-test and Pearson’s correlation. The findings revealed that AI technologies significantly enhance the personalization of fitness programmes, enabling users to achieve tailored workout structures and set personalized fitness goals. Participants reported increased physical activity levels and improved consistency in their fitness routines, highlighting the positive correlation between AI integration and individual fitness outcomes. Additionally, the study emphasizes the necessity of addressing ethical considerations, in terms of algorithmic partiality and data confidentiality, to ensure equitable access to AI-driven fitness solutions. It was recommended that fitness organizations and AI developers should focus on user education, engagement, and continuous improvement of user experience to maximize the benefits of AI technologies.
References
Boone, H.N. and Boone, D.A. (2012) Analyzing Likert Data. The Journal of Extension, 50, 1-5.
https://joe.org/joe/2012april/tt2.phpCoffey, M., Sutherland, R., & Coates, A. (2021). Gender Differences in Technology Adoption for Physical Activity: A Review of the Literature. Journal of Health Psychology, 26(7), 965-977.
Gagnon, M. P., Paré, G., Grenier, S., & Boucher, A. (2012). Telehealth Technologies: A Systematic Review of the Literature on Telehealth Implementation and Effectiveness. Journal of Health Services Research & Policy, 17(1), 16-24.
Global Wellness Institute. (2021). Global Wellness Trends: Insights and Innovations. Retrieved from https://globalwellnessinstitute.org
Greenhalgh, T., Robert, G., Macfarlane, F., Bate, P., & Kyriakidou, O. (2004). Diffusion of Innovations in Health Service Organizations: A Systematic Literature Review. Quality & Safety in Health Care, 13(2), 128-134. doi:10.1136/qshc.2003.009961
Jago, R., Edwards, M. J., & Kearney, C. (2016). The Role of Technology in the Promotion of Physical Activity in Children and Young People: A Systematic Review. International Journal of Behavioral Nutrition and Physical Activity, 13(1), 44.
Kwan, M. Y., Arbour-Nicitopoulos, K. P., & McCormack, G. R. (2020). The Impact of a Mobile Health Intervention on Health Behaviors and Fitness Outcomes in College Students: A Pilot Study. BMC Public Health, 20(1), 1102.
Liao, Y., Dong, M., & Liu, H. (2020). An Artificial Intelligence-Based Approach for the Personalized Health Management System. Computers in Biology and Medicine, 126, 104021.
McAvoy, C. (2023). "Wearables are getting smarter each year, and it is important we leverage these enhancements to motivate and inform our clients." American College of Sports Medicine Health & Fitness Journal, 27(1). https://journals.lww.com/acsm-healthfitness/pages/currenttoc.aspx
Morley, J., Pacuraru, G., & Mughal, F. (2019). Artificial Intelligence in Health: The Importance of Ethical Considerations. Health Informatics Journal, 25(3), 1024-1031. doi:10.1177/1460458218765054
Newsome, A. M. (2023). Clients are desiring instant feedback on their training so that they can adjust or modify to maximize outcomes and reduce injury. We are seeing this in individualized programming and in group-based classes." American College of Sports Medicine Health & Fitness Journal, 27(1). https://journals.lww.com/acsm-healthfitness/pages/currenttoc.aspx
Patton, M., O'Connor, R., & McMillan, C. (2016). A Framework for the Personalization of Fitness Programmes: Toward a Comprehensive Model. International Journal of Exercise Science, 9(4), 553-566.
Payne, H. E., Morrow, J. R., & Webber, C. (2015). The Impact of Wearable Activity Trackers on Physical Activity Behavior: A Systematic Review. Journal of Physical Activity & Health, 12(9), 1188-1194. doi:10.1123/jpah.12.9.1188
Petersen, S. J., & Gebhardt, W. A. (2017). The Role of Personalized Health Technology in Motivating Physical Activity: A Review of the Evidence. Journal of Physical Activity & Health, 14(5), 362-370. doi:10.1123/jpah.2016-0087
Rogers, E. M. (2003). Diffusion of Innovations (5th ed.). Free Press.
Srivastava, K., Joshi, A., & Sinha, D. (2020). Artificial Intelligence and Fitness: The Future of Personal Training. Journal of Fitness Research, 9(3), 45-52. doi:10.31741/jfr.v9i3.207