

Economics and Business
Quarterly Reviews
ISSN 2775-9237 (Online)


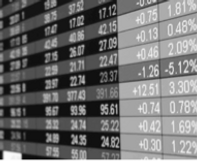




Published: 19 November 2019
Clustering Neural Network Analysis of Recreational Fisheries Management Strategies
Yeong Nain Chi
University of Maryland Eastern Shore, USA
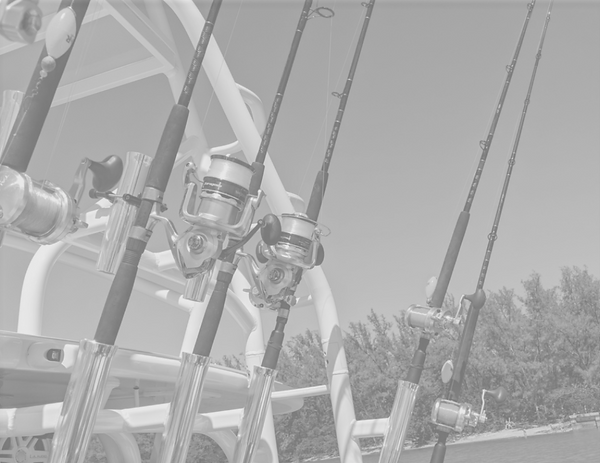
Download Full-Text Pdf

10.31014/aior.1992.02.04.164
Pages: 1238-1257
Keywords: Saltwater, Recreational Anglers, Preferences, Recreational Fisheries Management Strategies, Factor Analysis, Cluster Analysis, Discriminant Analysis, Multilayer Perceptron, Neural Network
Abstract
This study utilized data extracted from the 2013 National Saltwater Angler Survey to understand saltwater recreational anglers’ preferences toward recreational fisheries management strategies, to identify groups exhibiting common patterns of responses, and to examine the association between socio-demographic characteristics and the groups identified. Saltwater recreational anglers’ preferences toward recreational fisheries management strategies were examined through factor analysis which identified four reliable factors. Cluster analysis was employed to identify three prominent recreational angler groups. Statistical tests were employed to investigate the association between socio-demographic characteristics, including age, gender, income level, educational level, region of the respondent, and the identified recreational angler groups. The multilayer perceptron neural network model was utilized as a predictive model in deciding recreational anglers’ preferences toward recreational fishing management strategies. From an architectural perspective, it showed a 15-7-3 neural network construction. The results also revealed that fisheries habitat development and bag limit consideration were the greatest effect on how the recreational anglers’ preferences in terms of recreational fisheries management strategies. Results of this study may provide insight regarding the preferences toward recreational fisheries management strategies from saltwater recreational anglers as an indicator of potential participation and behavior of saltwater recreational fisheries management.
References
- Brinson, A. A. and Wallmo, K. 2013. Attitudes and preferences of salter recreational anglers: Report from the 2013 National Saltwater Angler Survey, Volume I. U.S. Department of Commerce, NOAA Technical Memorandum NMFS-F/SPO-135, 45p. Retrieved from: https://www.st.nmfs.noaa.gov/Assets/economics/ documents /rec-attitudes/Rec%20Attitudes%20Report%20TM%20135.pdf
- Chauvin, Y. and Rumelhart, D. E. (Eds.). (1995). Developments in connectionist theory. Back-propagation: Theory, architectures, and applications. Hillsdale, NJ: Lawrence Erlbaum Associates, Inc.
- Child, D. (2006). The essentials of factor analysis (Third Ed.). New York, NY: Continuum International Publishing Group.
- Churchill, G. A., Jr. and Iacobucci, D. (2005). Marketing research: methodological foundations (Ninth Ed.). Mason, OH: Thomson/South-Western.
- Cronbach, L. J. (1951). Coefficient alpha and the internal structure of tests. Psychometrika16(3), 297-334.
- Driver, B. L. and Knopf, R. C. (1976). Temporary escape: one product of sport fisheries management. Fisheries 1(2), 2-19.
- Fisher, R. A. (1936). The use of multiple measurements in taxonomic problems. Annals of Eugenics 7, 179-188.
- Forgy, E. W. (1965). Cluster analysis of multivariate data: efficiency versus interpretability of classifications. Biometrics 21, 768–769.
- Gardner, M. W. and Dorling, S. R. (1998). Artificial neural networks (the multilayer perceptron) - A review of applications in the atmospheric sciences. Atmospheric Environment 32(14), 2627-2636.
- Gerbing, D. W. and Anderson, J. C. (1988). An updated paradigm for scale development incorporating unidimensionality and its assessment. Journal of Marketing Research 25, 186-192.
- Hilborn, R. (2007). Moving to sustainability by learning from successful fisheries. Ambio: A Journal of the Human Environment 36(4), 296-303.
- IBM. (2019). IBM SPSS neural networks 26. Armonk, NY: IBM Corporation.
- Ihde, T. F., Wilberg, M. J., Loewensteiner, D. A., Secor, D. H. and Miller, T. J. (2010). The increasing importance of marine recreational fishing in the U.S.: challenges for management. Fisheries Research 108, 268-276.
- MacQueen, J. (1967) Some Methods for Classification and Analysis of Multivariate Observations. Proceedings of the 5th Berkeley Symposium on Mathematical Statistics and Probability 1, 281-297.
- Manel S, Dias, J. M., Buckton, S. T., and Ormerod, S. J. (1999). Alternative methods for predicting species distribution: an illustration with Himalayan river birds. Journal of Applied Ecology 36, 734–747.
- National Marine Fisheries Service. (2015). National saltwater recreational fisheries policy 2015. U.S. Dept. of Commerce, NOAA, 8p. Retrieved from: http://www.nmfs.noaa.gov/sfa/management/recreational/documents/noaa_recfish_policy.pdf.
- National Marine Fisheries Service. (2017). Marine recreational information program strategic plan: 2017-2022. U.S. Dept. of Commerce, NOAA, 27p. Retrieved from: https://www.st.nmfs.noaa.gov/Assets/recreational/ documents/mrip-strategic-plan/2017_Strategic_Plan_forPublicComment.pdf.
- National Marine Fisheries Service. (2017). Fisheries Economics of the United States, 2015: Economics and Socio-cultural Status and Trend Series. U.S. Dept. of Commerce, NOAA Technical Memorandum NMFS-F/SPO-170, 247p. Retrieved from: http://www.st.nmfs.noaa.gov/Assets/economics/publications/FEUS/FEUS-2015/Report-Chapters/FEUS%202015-AllChapters_Final.pdf
- Rumelhart. D. E., Hinton. G. E. and Williams, R. J. (1986). Learning internal representations by error propagation. In D. E. Rumelhart, J. L. McClelland and the PDP research group, (Eds). Parallel distributed processing: explorations in the microstructure of cognition, Volume 1. Cambridge, MA: MIT Press.
- Schroeder, S. A., Fulton, D. C., Nemeth, M. L., Sigurdson, R. E. and Walsh, R. J. (2008). Fishing in the neighborhood: understanding motivations and constraints for angling among Minneapolis–St. Paul, Minnesota metro residents. American Fisheries Society Symposium 67, Bethesda, Maryland.
- Sheela, K. G. and Deepa, S. N. (2013). Review on Methods to Fix Number of Hidden Neurons in Neural Networks. Mathematical Problems in Engineering Volume 2013, Article ID 425740, 11p.
- Suryanarayana, I., Braibanti, A., Rao, R. S., Ramamc, V. A., Sudarsan, D. and Rao, G. N. (2008). Neural networks in fisheries research. Fisheries Research92, 115–139.
- Tabatchnick, B. G. and Fidell, L. S. (2013). Using multivariate statistics (Sixth Ed.). Boston: Pearson Education, Inc.
- The Commission on Saltwater Recreational Fisheries Management. (2014). A vision for managing America’s saltwater recreational fisheries. The Commission on Saltwater Recreational Fisheries Management, 16p. Retrieved from: http://asafishing.org/wp-content/uploads/Marine_Visioning_Report_Spring_2014.pdf.
- Tseng, Y. P., Huang, T. C. and Ditton, R. (2012). Developing a longitudinal perspective on the human dimensions of recreational fisheries. Journal of Coastal Research28, 1418-1425.